Advances in Machine Learning and Statistical Analysis of Geographical Data
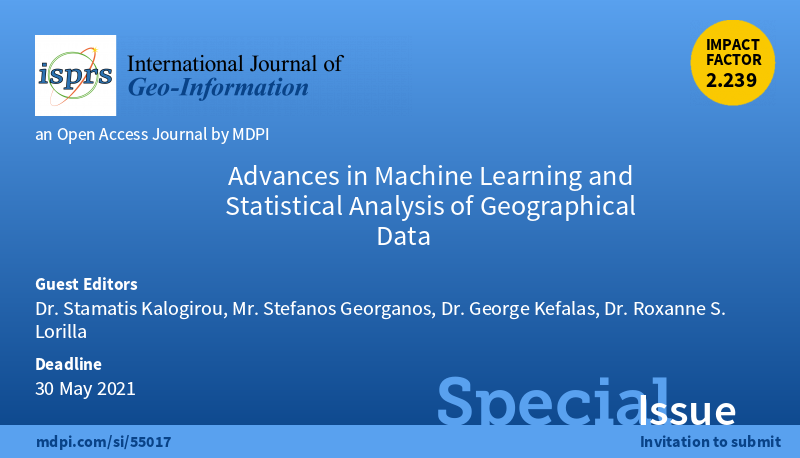
Dear Colleagues,
Key technologies that enable the digital transformation of public institutions and private companies have been in the spotlight for some years now. Theories related to Artificial Intelligence, data science, and machine learning have a long history. The recent evolution of information technology and communications infrastructure (cheaper and more powerful machines, fast network connections), along with high-level open source programming languages (such as R and Python), enables extensive application of the above technologies to real world data. The datafication of more aspects of human life, machine performance, and the environment on earth and beyond enables us to expand the spectrum of data driven decisions.
To address existing and new challenges of increased complexity, we must always evolve, and so should our analysis. The scarcity of resources and the need for efficiency and fast results leave less room for mistakes. This creates the need to achieve more trustworthy results while increasing their robustness. In geography, we have known for decades that location matters. Established techniques such as local models have addressed the issue of spatial heterogeneity and allowed for the discovery of local processes hidden in traditional models. However, modern algorithms related to Artificial Intelligence, machine learning, and classification of geospatial data are not always “location-aware” even though they manage to achieve more robust results compared to traditional methods.
This Special Issue aims to capture the state-of-the-art theories and applications of machine learning and advanced statistics used to analyze spatial data, including big spatial data. The use of advanced methods is linked to appropriate theoretical frameworks (properly acknowledging previous work in the topic) and ethical/responsible use of AI, while encouraging transparency and reproducibility. We look forward to receiving your contribution and we trust this Special Issue will become an excellent reference for future advancements in the domain.
We particularly welcome developments related but not limited to:
- Spatial statistics;
- Machine learning applications on spatial data;
- Machine learning for remote sensing applications;
- Improvements on existing AI models tailored to spatial data analysis;
- Proposing new methods to couple geographical information with AI solutions;
- Innovative ways to fuse multisource spatial data with AI algorithms;
- Comparative analysis between machine learning and traditional geostatistics to solve geographical problems;
- Landscape assessment and ecosystem modeling;
- Spatial dynamics of environmental processes;
- Assessing socioecological systems;
- Revealing drivers of ecosystem services, landscape changes/transitions, etc.;
- Advanced machine learning algorithms/techniques for object-based image analysis.
Dr. Stamatis Kalogirou
Mr. Stefanos Georganos
Dr. George Kefalas
Dr. Roxanne S. Lorilla
Guest EditorsMore information is available here https://www.mdpi.com/journal/ijgi/special_issues/MachineLearning_Statistical